Customer lifetime value: What’s new?
How can retailers use technology to improve their CLV to boost the bottom line? Experts from Amperity reveal all.
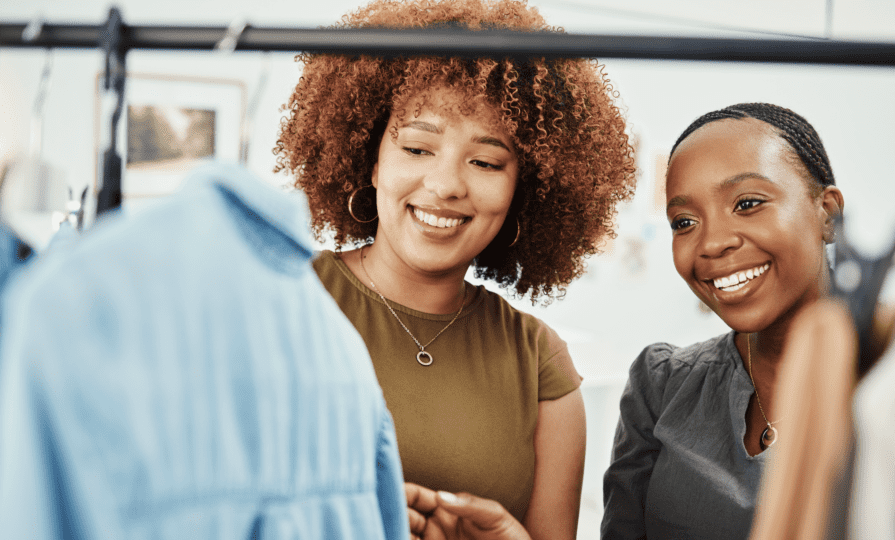
Customer Lifetime Value (CLV) represents a crucial metric that measures a customer’s revenue potential over their entire relationship with a retail brand. In many ways, it is the “north” star metric for customer-centric brands that want to build long-term profitable relationships with their customers across the entire omnichannel journey. So how can retailers use technology to improve their CLV to boost the bottom line? Experts from Amperity reveal all.
The benefit of knowing your customers’ CLV
Knowing your customers’ CLV allows brands to evaluate long-term profitability, guide marketing decisions, prioritise high-value customer segments, and optimise resource allocation. According to Margaret Gorin, Head of Value Advisory at Amperity, brands that don’t operate based on CLV tend to overly focus on transactional metrics and aggregate metrics and/or channel metrics.
“However those KPIs can lead to myopic decisions that tend to favor short-term growth over long-term profitability. However, calculating CLV is often easier said than done,” she says.
Some challenges of calculating CLV include data quality and integration, accurately defining the lifetime period, and keeping pace with evolving customer behaviors.
“To navigate some of these challenges, brands should consider a customer data platform (CDPs). A data system that can unify and cleanse customer data from multiple touchpoints, will help combat inaccurate or fragmented data that hinders CLV calculations,” she says.
With the right systems in place, brands should then take the following steps into consideration:
– Accurately unify the full transaction history across every channel for each customer: This step is critical in understanding your customers transaction history and informing predictions.
– Determine the time horizon or “lifetime” across which you want to calculate CLV: This will depend on each brand’s typical inter-purchase cycle and goals. For example, mattress brands may calculate CLV over a 10 year period, while grocery stores may calculate CLV over the course of just 1 year.
– Determine whether you want CLV to be returns-netting, and if you want to calculate based on net profit or gross revenue: Most retail brands opt for returns-netting CLV so that they can differentiate customers who frequently buy and return from customers who are truly high value.
– Calculate CLV on each customer, then stack-rank your customers based on their CLV and assign them to different value tiers: The top 1% of customers in terms of CLV, the top 5%, top 10%, etc. This will allow you to easily compare your highest value customers to lower value customers and segment your marketing based on value tier.
– Track CLV on a cohort basis to measure the longitudinal behavior of customers who were acquired at different points in time: This can be done at both an overall level as well as at a segment level.
– Ensure your processes, org structure and operating model are designed to measure and maximise CLV: It’s critical that your data and technology infrastructure support continuous measurement.
– As your CLV calculation and tracking capabilities mature, consider building predictive CLV models as an additional analytical & segmentation tool: CDPs with sophisticated ML-based techniques can predict each customer’s future CLV, even if they’ve only just made their first purchase.
How AI is revolutionising deeper customer connections
Artificial intelligence (AI) is revolutionising the software solutions that retailers use for marketing, particularly around identifying, understanding and connecting with their customers. According to Derek Slager, CTO and co-founder of Amperity, early iterations of AI within these tools may have been slow to meet business needs. However, new advances in AI and machine learning (ML) have unlocked capabilities once thought impossible.
“Retailers can leverage AI’s capabilities within customer data platforms (CDPs) to create unified customer profiles that improve customer lifetime value and capture unrealised consumer demand,” he says. “By using AI and CDPs, brands can achieve a 360-degree view of their customers, enabling them to upsell and cross-sell more effectively.”
For example, when a customer purchases a laptop, there is an opportunity for the retailer to promote and sell accessories such as computer bags, external hard drives and wireless mice.
In addition, with unified customer profiles, brands have a clear understanding of who their high-value customers are and can identify additional look-alikes to target. For example, if a long-term customer consistently purchases new running shoes and new socks every six months, there may be an opportunity to target other customers that purchase more than one pair of running shoes a year to motivate them to make additional purchase of items such as energy gels, socks or other gear.
“With a CDP, it is much easier for brands to use data to develop these types of targeted and tailored campaigns, leading to better retention, higher sales and bigger profits,” he adds.
Map customer lifecycles to improve lifetime value
Slager explains how with a CDP, brands can ingest raw customer data across many sources – from online and in-store interactions to loyalty programs, email engagements and financial systems. Once the CDP has captured that data, it uses ML to resolve identities even when records lack unique identifiers across systems.
“AI connects essential customer information, including demographics, loyalty, email engagement, and product purchase data, allowing brands with this software to collect richer, cleaner data. This in turn improves ML modeling performance. As a result, brands can use this insight to understand customer lifetime value, enabling them to make strategic decisions related to marketing, customer acquisition and customer retention,” he adds.
For example, say a cosmetic company is interested in calculating the lifetime value for a particular customer. AI algorithms, such as machine learning models, can analyze vast amounts of data to predict customers’ future behaviors.
The software can analyse their past purchases, preferences for online or in-store shopping, frequency of purchases and more, all of which help to more accurately predict their future buying habits. And through these insights, marketers can more effectively reach customers with more relevant ads and product offers, increasing retention and driving sales.
Predictive analytics and customer lifetime value
Predictive analytics offers brands a powerful tool to boost customer retention and improve the customer experience. Barry Padgett, CEO at Amperity says CLV, while essential to any business, requires predictive analytics to indicate how much revenue companies can expect from each customer over the span of their business relationships.
“With the key insights generated from this metric, brands can better understand which customers are spending money, enabling brands to target them with tailored advertising and marketing that can drive business, increase retention rate and improve the customer experience,” he explains.
According to Padgett, this calculation, however, includes many components and it’s key that the data foundation in place is accurate in order to generate a precise CLV. For example, many brands struggle to unify their offline transactions with digital interactions, and most are misidentifying as much as a quarter of their customers, meaning calculations will be inaccurate.
“It is critical for brands to have a clean data foundation to derive more value from their best customers, while still identifying new customers who share the same attributes and potential spending power. Unified customer profiles and audience segmentation ensure marketers reach the right customers at the right time through the most impactful channel,” he adds.
“When brands put customers and their needs at the center of their business, they are bound to succeed. But when they can truly understand their customers based on access to clean, accurate data, they can be unstoppable and calculate accurate CLVs that offer invaluable insights into their business. By understanding customers’ shopping habits, preferences and how they interact with ads, brands can tailor specific advertisements to each customer, improving the customer experience and increasing retention. Through this, brands can drive revenue and continue to meet the needs of their customers,” he concludes.
About Amperity
Amperity delivers the data confidence brands need to unlock growth by truly knowing their customers. With Amperity, brands can build a first-party data foundation to fuel customer acquisition and retention, personalise experiences that build loyalty, and manage privacy compliance. Using patented AI and ML methods, Amperity stitches together all customer interactions to build a unified view that seamlessly connects to marketing and technology tools. More than 400 brands worldwide rely on Amperity to turn data into business value, including Alaska Airlines, DICK’S Sporting Goods, Endeavour Drinks, Planet Fitness, Seattle Sounders FC, Under Armour and Wyndham Hotels & Resorts. For more information, visit amperity.com or follow us on Linkedin, Twitter, Facebook and Instagram.